Table Of Content
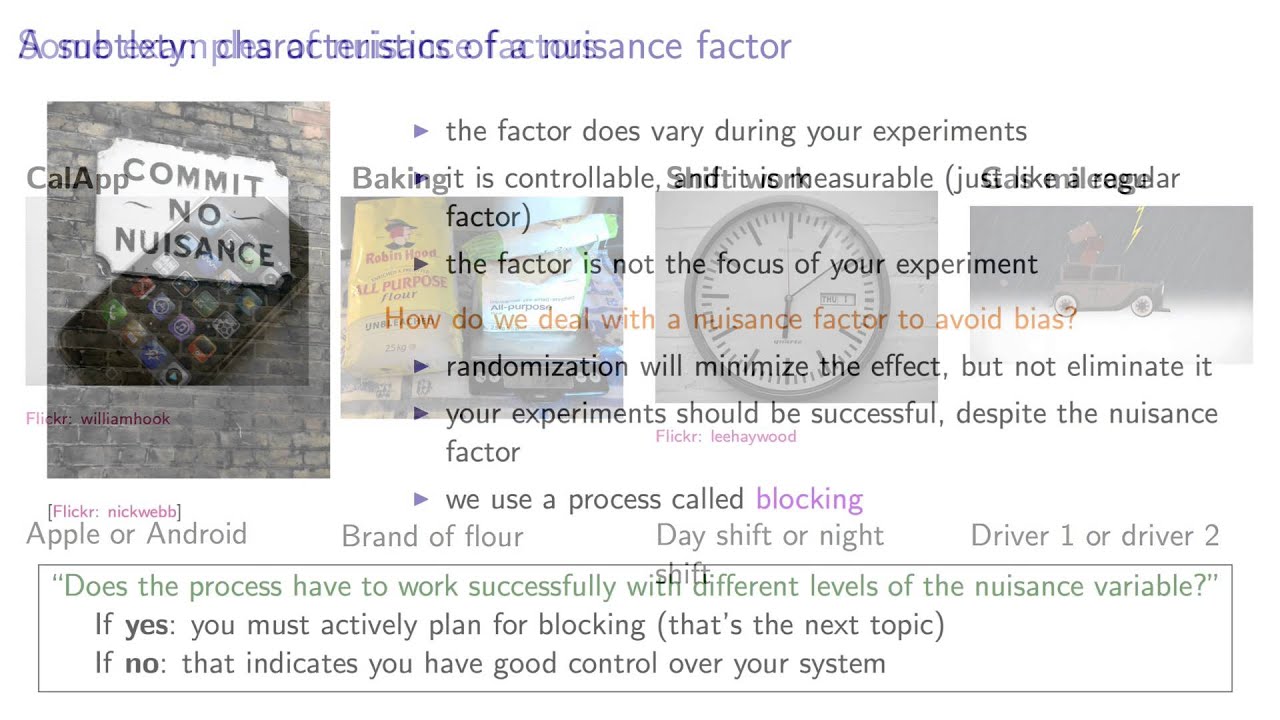
All ordered pairs occur an equal number of times in this design. It is balanced in terms of residual effects, or carryover effects. Together, you can see that going down the columns every pairwise sequence occurs twice, AB, BC, CA, AC, BA, CB going down the columns. The combination of these two Latin squares gives us this additional level of balance in the design, than if we had simply taken the standard Latin square and duplicated it. Crossover designs use the same experimental unit for multiple treatments. The common use of this design is where you have subjects (human or animal) on which you want to test a set of drugs -- this is a common situation in clinical trials for examining drugs.
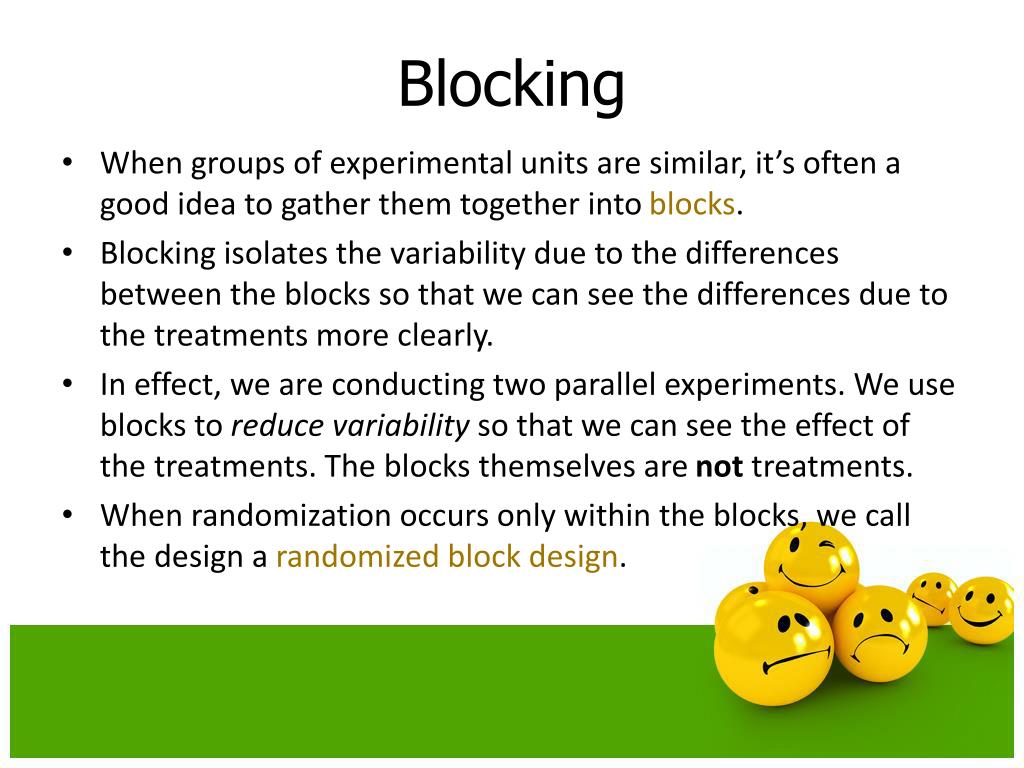
Design of Experiments – Blocking and Full Factorial Experimental Design Plans
This type of experimental design is surprisingly powerful and often results in a high probability to create a near optimal design. If you look at how we have coded data here, we have another column called residual treatment. For the first six observations, we have just assigned this a value of 0 because there is no residual treatment. But for the first observation in the second row, we have labeled this with a value of one indicating that this was the treatment prior to the current treatment (treatment A). In this way the data is coded such that this column indicates the treatment given in the prior period for that cow.
Design of Experiments with R. Building 2^k Factorial Designs by Roberto Salazar - Towards Data Science
Design of Experiments with R. Building 2^k Factorial Designs by Roberto Salazar.
Posted: Tue, 03 Dec 2019 05:32:29 GMT [source]
Error
In this case, we have different levels of both the row and the column factors. Again, in our factory scenario, we would have different machines and different operators in the three replicates. In other words, both of these factors would be nested within the replicates of the experiment. For instance, we might do this experiment all in the same factory using the same machines and the same operators for these machines. The first replicate would occur during the first week, the second replicate would occur during the second week, etc.
ANOVA: Yield versus Batch, Pressure
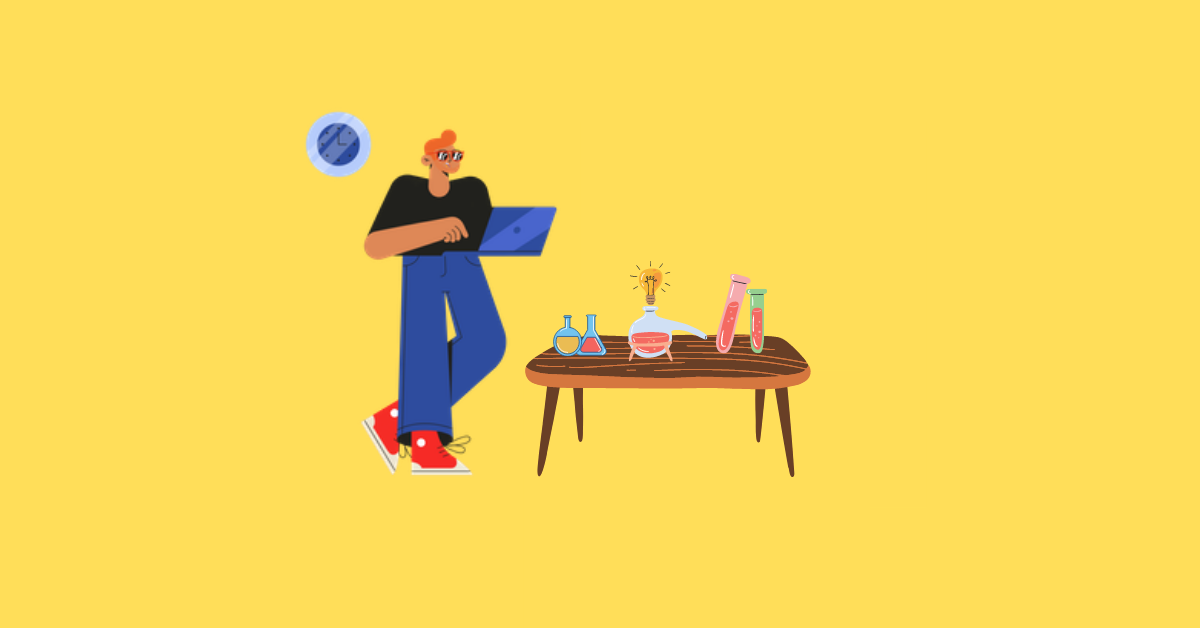
The fundamental idea of blocking can be extended to more dimensions. However, the full use of multiple blocking variables in a complete block design usually requires many experimental units. Latin Square design can be useful when we want to achieve blocking simultaneously in two directions with a limited number of experimental units.
In addition, considering the correlation between the image and prompt, AMFF-Net compares the semantic features from text encoder and image encoder to evaluate the text-to-image alignment. We carry out extensive experiments on three AGI quality assessment databases, and the experimental results show that our AMFF-Net obtains better performance than nine state-of-the-art blind IQA methods. The results of ablation experiments further demonstrate the effectiveness of the proposed multi-scale input strategy and AFF block. The ideal experimental design is the randomized controlled double-blind experiment. Since the first three columns contain some pairs more than once, let's try columns 1, 2, and now we need a third...how about the fourth column. If you look at all possible combinations in each row, each treatment pair occurs only one time.
A design of experiments Cyber–Physical System for energy modelling and optimisation in end-milling machining - ScienceDirect.com
A design of experiments Cyber–Physical System for energy modelling and optimisation in end-milling machining.
Posted: Tue, 18 Oct 2022 08:16:44 GMT [source]
Abstract
The next thing you need to do after you determine your blocking factors is allocate your observations into blocks. To simplify things, we will assume that you have one main blocking factor that you want to balance over. First the individual observational units are split into blocks of observational units that have similar values for the key variables that you want to balance over. After that, the observational units from each block are evenly allocated into treatment groups in a way such that each treatment group is allocated similar numbers of observational units from each block. With the increasing maturity of the text-to-image and image-to-image generative models, AI-generated images (AGIs) have shown great application potential in advertisement, entertainment, education, social media, etc.
How does blocking work in experimental design?
Therefore we partition our subjects by gender and from there into age classes. Thus we have a block of subjects that is defined by the combination of factors, gender and age class. The final step in the blocking process is allocating your observations into different treatment groups. All you have to do is go through your blocks one by one and randomly assign observations from each block to treatment groups in a way such that each treatment group gets a similar number of observations from each block. The first step of implementing blocking is deciding what variables you need to balance across your treatment groups.
Another way to think about this is that a complete replicate of the basic experiment is conducted in each block. In this case, a block represents an experimental-wide restriction on randomization. Back to the hardness testing example, the experimenter may very well want to test the tips across specimens of various hardness levels. To conduct this experiment as a RCBD, we assign all 4 tips to each specimen. Sometimes several sources of variation are combined to define the block, so the block becomes an aggregate variable. Consider a scenario where we want to test various subjects with different treatments.
Video 1: Experimental Design Examples
In Design of Experiments, blocking involves recognizing uncontrolled factors in an experiment–for example, gender and age in a medical study–and ensuring as wide a spread as possible across these nuisance factors. Let’s take participant gender in a simple 3-factor experiment as an example. Here is an actual data example for a design balanced for carryover effects. In this example the subjects are cows and the treatments are the diets provided for the cows. Using the two Latin squares we have three diets A, B, and C that are given to 6 different cows during three different time periods of six weeks each, after which the weight of the milk production was measured. We have not randomized these, although you would want to do that, and we do show the third square different from the rest.
Note that Case 2 may also be flipped where you might have the same machines, but different operators. Where F stands for “Full” and R stands for “Reduced.” The numerator and denominator degrees of freedom for the F statistic is \(df_R - df_F\) and \(df_F\) , respectively. Remember, the hardness of specimens (coupons) is tested with 4 different tips. Imagine an extreme scenario where all of the athletes that are running on turf fields get allocated into one group and all of the athletes that are running on grass fields are allocated into the other group.
If you think a variable could influence the response, you should block on that variable. Here is a plot of the least square means for treatment and period. We can see in the table below that the other blocking factor, cow, is also highly significant.
In some disciplines, each block is called an experiment (because a copy of the entire experiment is in the block) but in statistics, we call the block to be a replicate. This is a matter of scientific jargon, the design and analysis of the study is an RCBD in both cases. A block is characterized by a set of homogeneous plots or a set of similar experimental units. In agriculture a typical block is a set of contiguous plots of land under the assumption that fertility, moisture, weather, will all be similar, and thus the plots are homogeneous. Many industrial and human subjects experiments involve blocking, or when they do not, probably should in order to reduce the unexplained variation. Here are the main steps you need to take in order to implement blocking in your experimental design.
What you also want to notice is the standard error of these means, i.e., the S.E., for the second treatment is slightly larger. The fact that you are missing a point is reflected in the estimate of error. You do not have as many data points on that particular treatment. Basic residual plots indicate that normality, constant variance assumptions are satisfied. Therefore, there seems to be no obvious problems with randomization. These plots provide more information about the constant variance assumption, and can reveal possible outliers.
What we now realize is that two blocking factors is not enough! It looks like day of the week could affect the treatments and introduce bias into the treatment effects, since not all treatments occur on Monday. We want a design with 3 blocking factors; machine, operator, and day of the week.
No comments:
Post a Comment